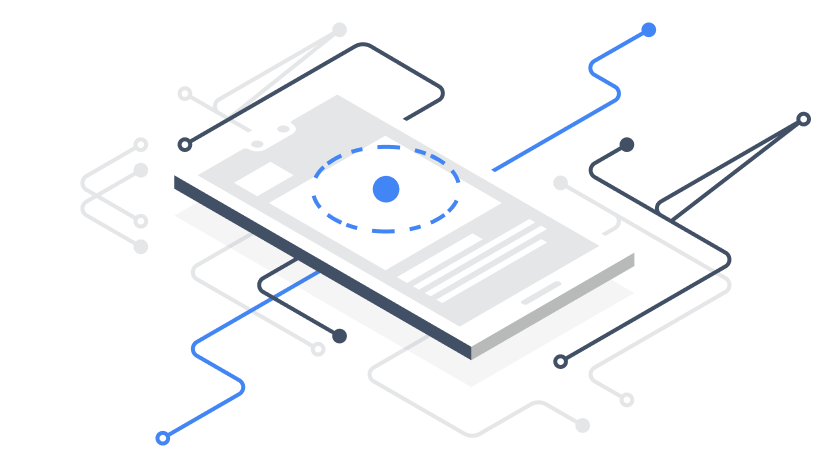

Learn Machine Learning
- Google open sources tools to support AI model developmenttechcrunch.com Google open sources tools to support AI model development | TechCrunch
Google is launching Jetstream, a new engine to run generative AI models, and MaxDiffusion, a collection of reference implementations of various diffusion models.
- Finetune LLMs on your own consumer hardware using tools from PyTorch and Hugging Face ecosystempytorch.org Finetune LLMs on your own consumer hardware using tools from PyTorch and Hugging Face ecosystem
We demonstrate how to finetune a 7B parameter model on a typical consumer GPU (NVIDIA T4 16GB) with LoRA and tools from the PyTorch and Hugging Face ecosystem with complete reproducible Google Colab notebook.
- Understanding GPU Memory 2: Finding and Removing Reference Cyclespytorch.org Understanding GPU Memory 2: Finding and Removing Reference Cycles
This is part 2 of the Understanding GPU Memory blog series. Our first post Understanding GPU Memory 1: Visualizing All Allocations over Time shows how to use the memory snapshot tool. In this part, we will use the Memory Snapshot to visualize a GPU memory leak caused by reference cycles, and then l...
- PyTorch: Compiling NumPy code into C++ or CUDA via torch.compilepytorch.org Compiling NumPy code into C++ or CUDA via torch.compile
Quansight engineers have implemented support for tracing through NumPy code via torch.compile in PyTorch 2.1. This feature leverages PyTorch’s compiler to generate efficient fused vectorized code without having to modify your original NumPy code. Even more, it also allows for executing NumPy code...
- Introduction to Kernel Methods for Machine Learning
Kernel methods give a systematic and principled approach to training learning machines and the good generalization performance achieved can be readily justified using statistical learning theory or Bayesian arguments. We describe how to use kernel methods for classification, regression and novelty detection and in each case we find that training can be reduced to optimization of a convex cost function.
- The Kernel Cookbook: Advice on Covariance functions
If you've ever asked yourself: "How do I choose the covariance function for a Gaussian process?" this is the page for you. Here you'll find concrete advice on how to choose a covariance function for your problem, or better yet, make your own.
- An Intuitive Tutorial to Gaussian Processes Regression
This tutorial aims to provide an intuitive understanding of the Gaussian processes regression. Gaussian processes regression (GPR) models have been widely used in machine learning applications because of their representation flexibility and inherent uncertainty measures over predictions.
- [Resource] Understanding UMAP - Google PAIRpair-code.github.io Understanding UMAP
UMAP is a new dimensionality reduction technique that offers increased speed and better preservation of global structure.
Has nice interactive examples and UMAP vs t-SNE
- [Resource] MIT OpenCourseWare: Introduction To Machine Learningocw.mit.edu Introduction to Machine Learning | Electrical Engineering and Computer Science | MIT OpenCourseWare
This course introduces principles, algorithms, and applications of machine learning from the point of view of modeling and prediction. It includes formulation of learning problems and concepts of representation, over-fitting, and generalization. These concepts are exercised in supervised learning an...
- DuckAI - An open-source ML research community
https://duckai.org/
cross-posted from: https://lemmy.intai.tech/post/134262
> DuckAI is an open and scalable academic lab and open-source community working on various Machine Learning projects. Our team consists of researchers from the Georgia Institute of Technology and beyond, driven by our passion for investigating large language models and multimodal systems. > > Our present endeavors concentrate on the development and analysis of a variety of dataset projects, with the aim of comprehending the depth and performance of these models across diverse domains. > > Our objective is to welcome people with a variety of backgrounds to cutting-edge ML projects and rapidly scale up our community to make an impact on the ML landscape. > > We are particularly devoted to open-sourcing datasets that can turn into an important infrastructure for the community and exploring various ways to improve the design of foundation models.
- [Resource] Style Guide for Python Code: PEP 8peps.python.org PEP 8 – Style Guide for Python Code | peps.python.org
Python Enhancement Proposals (PEPs)
- [Resource] MIT OpenCourseWare: Statistical Learning Theoryocw.mit.edu Topics in Statistics: Statistical Learning Theory | Mathematics | MIT OpenCourseWare
The main goal of this course is to study the generalization ability of a number of popular machine learning algorithms such as boosting, support vector machines and neural networks. Topics include Vapnik-Chervonenkis theory, concentration inequalities in product spaces, and other elements of empiric...
- [Resource] MIT OpenCourseWare: Mathematics Of Machine Learningocw.mit.edu Mathematics of Machine Learning | Mathematics | MIT OpenCourseWare
Broadly speaking, Machine Learning refers to the automated identification of patterns in data. As such it has been a fertile ground for new statistical and algorithmic developments. The purpose of this course is to provide a mathematically rigorous introduction to these developments with emphasis on...
Broadly speaking, Machine Learning refers to the automated identification of patterns in data. As such it has been a fertile ground for new statistical and algorithmic developments. The purpose of this course is to provide a mathematically rigorous introduction to these developments with emphasis on methods and their analysis.
- [Resource] Durham University Materials for COMP3547 (Deep Learning) and COMP3667 (Reinforcement Learning) from Dr. Robert Lieckgithub.com GitHub - cwkx/materials: lecture materials
lecture materials. Contribute to cwkx/materials development by creating an account on GitHub.
Includes lectures, lecture notes and assignments.
Lectures for Deep Learning: https://www.youtube.com/playlist?list=PLMsTLcO6etti_SObSLvk9ZNvoS_0yia57
Lectures for Reinforcement Learning: https://www.youtube.com/playlist?list=PLMsTLcO6ettgmyLVrcPvFLYi2Rs-R4JOE
- [Resource] Rules of Machine Learning from Google
A good set of best practices for deployment that isn't language-specific
- [Resource] Coding Practices for Python/MLgithub.com GitHub - zedr/clean-code-python: :bathtub: Clean Code concepts adapted for Python
:bathtub: Clean Code concepts adapted for Python. Contribute to zedr/clean-code-python development by creating an account on GitHub.
Coding nowadays is a big part of ML and while it's important that the model works well, it's also important that the code is written properly too.
Link is the general python version, ML-specific version here: https://github.com/davified/clean-code-ml
Video version: https://bit.ly/2yGDyqT
- [Resource] Tutorial: Image Recognition with CNN in Matlab
Introduces neural networks, the convolution operation, a few critical machine learning concepts and some state-of-the-art CNN models. Includes a hands-on Matlab tutorial (and code) demonstrating the model configuration, training process, and performance evaluation using the MNIST dataset.
- [Resource] Tutorial: State of Charge Estimation with EKF and SVSF in Matlab
This tutorial describes the process for the state of charge (SOC) estimation of Li-Ion cells using an equivalent circuit model. It helps students create and run a SOC estimation strategy based on the 3rd-order R-RC model in MATLAB-Simulink. The tutorial starts with a general overview of state estimation using the extended Kalman filter (EKF) and the novel smooth variable structure filter (SVSF) method.
- [Resource] Standford University Cheat Sheets for ML (web version)
I'm not sure if I'd call a 10+ page pdf a "cheat sheet" but they are good resources
- Mathematics for Neural Networks
Can't say I agree with all of this 100% (I'd put backpropagation in the math side, add in model evaluation, remove convex optimization, etc) plus it's kind of an oversimplification but the basics are there
- [Resource] Materials from CORNELL CS4780/CS5780: Machine Learning for Intelligent Systems
Lecture notes: https://www.cs.cornell.edu/courses/cs4780/2018fa/syllabus/
Recorded lectures: https://www.youtube.com/playlist?list=PLl8OlHZGYOQ7bkVbuRthEsaLr7bONzbXS
- My LLM CLI tool now supports self-hosted language models via pluginssimonwillison.net My LLM CLI tool now supports self-hosted language models via plugins
LLM is my command-line utility and Python library for working with large language models such as GPT-4. I just released version 0.5 with a huge new feature: you can now …
- Introduction to Domain Adaptation for Neural Networksmachinelearning.apple.com Bridging the Domain Gap for Neural Models
Deep neural networks are a milestone technique in the advancement of modern machine perception systems. However, in spite of the exceptional…
- The standardization fallacy: the importance of variancewww.nature.com The standardization fallacy - Nature Methods
“We demand rigidly defined areas of doubt and uncertainty!” —D. Adams
- OpenChat_8192 - The first model to beat 100% of ChatGPT-3.5
cross-posted from: https://lemmy.intai.tech/post/40699
> ## Models > - opnechat > - openchat_8192 > - opencoderplus > > ## Datasets > - openchat_sharegpt4_dataset > > ## Repos > - openchat > > ## Related Papers > - LIMA Less is More For Alignment > - ORCA > > > ### Credit: > Tweet > > ### Archive: > @Yampeleg > The first model to beat 100% of ChatGPT-3.5 > Available on Huggingface > > 🔥 OpenChat_8192 > > 🔥 105.7% of ChatGPT (Vicuna GPT-4 Benchmark) > > Less than a month ago the world witnessed as ORCA [1] became the first model to ever outpace ChatGPT on Vicuna's benchmark. > > Today, the race to replicate these results open-source comes to an end. > > Minutes ago OpenChat scored 105.7% of ChatGPT. > > But wait! There is more! > > Not only OpenChat beated Vicuna's benchmark, it did so pulling off a LIMA [2] move! > > Training was done using 6K GPT-4 conversations out of the ~90K ShareGPT conversations. > > The model comes in three versions: the basic OpenChat model, OpenChat-8192 and OpenCoderPlus (Code generation: 102.5% ChatGPT) > > This is a significant achievement considering that it's the first (released) open-source model to surpass the Vicuna benchmark. 🎉🎉 > > - OpenChat: https://huggingface.co/openchat/openchat > - OpenChat_8192: https://huggingface.co/openchat/openchat_8192 (best chat) > - OpenCoderPlus: https://huggingface.co/openchat/opencoderplus (best coder) > > - Dataset: https://huggingface.co/datasets/openchat/openchat_sharegpt4_dataset > > - Code: https://github.com/imoneoi/openchat > > Congratulations to the authors!! > > --- > > [1] - Orca: The first model to cross 100% of ChatGPT: https://arxiv.org/pdf/2306.02707.pdf > [2] - LIMA: Less Is More for Alignment - TL;DR: Using small number of VERY high quality samples (1000 in the paper) can be as powerful as much larger datasets: https://arxiv.org/pdf/2305.11206
- GitHub - microsoft/Data-Science-For-Beginners: 10 Weeks, 20 Lessons, Data Science for All!
cross-posted from: https://lemmy.intai.tech/post/24579
> https://github.com/microsoft/Data-Science-For-Beginners
- Emerging Architectures for LLM Applications | Andreessen Horowitza16z.com Emerging Architectures for LLM Applications | Andreessen Horowitz
A reference architecture for the LLM app stack. It shows the most common systems, tools, and design patterns used by AI startups and tech companies.
- Mathematical Foundations of Machine Learning
cross-posted from: https://lemmy.intai.tech/post/21511
> https://skim.math.msstate.edu/LectureNotes/Machine_Learning_Lecture.pdf
- Neural Network Interactive Browser App: Tensorflow Playgroundplayground.tensorflow.org Tensorflow — Neural Network Playground
Tinker with a real neural network right here in your browser.
- MPT-30B-Chat - a Hugging Face Space by mosaicml
cross-posted from: https://lemmy.intai.tech/post/17993
> https://huggingface.co/spaces/mosaicml/mpt-30b-chat
- 101 fundamentals for aspiring the model makers
cross-posted from: https://lemmy.intai.tech/post/18067
> https://twitter.com/FrnkNlsn/status/1520585408215924736 > > https://www.researchgate.net/publication/327304999_An_Elementary_Introduction_to_Information_Geometry > > https://www.researchgate.net/publication/357097879_The_Many_Faces_of_Information_Geometry > > https://franknielsen.github.io/IG/index.html > > https://franknielsen.github.io/GSI/ > > https://www.youtube.com/watch?v=w6r_jsEBlgU&embeds_referring_euri=https%3A%2F%2Ftwitter.com%2F&source_ve_path=MjM4NTE&feature=emb_title
- [Resource] Good collection of introductions to topics for stats and machine learning: Nature Methods' Points of Significancewww.nature.com Points of Significance | Statistics for Biologists
A collection of articles from the publisher of Nature that discusses statistical issues biologists should be aware of and provides practical advice to improve the statistical rigor and reproducibility of their work.
From Nature.com - Statistics for Biologists. A series of short articles that are a nice introduction to several topics and because the audience is biologists, the articles are light on math/equations.
- [Discussion] What are your favourite tools for searching for info and why?
Bing, ChatGPT, etc.
- On sourcing for benchmark datasets: Will the Real Iris Data Please Stand Up?
This paper highlights an issue that many people don't think about. Fyi when trying to compare or reproduce results, always try to get the dataset from the same source as the original author and scale it in the same way. Unfortunately, many authors assume the scaling is obvious and don't include it but changes in scaling can lead to very different results.
- [Repost Q] Fast python convolution along one axis only
Not OP. This question is being reposted to preserve technical content removed from elsewhere. Feel free to add your own answers/discussion.
I have two 2-D arrays with the same first axis dimensions. In python, I would like to convolve the two matrices along the second axis only. I would like to get C below without computing the convolution along the first axis as well.
``` import numpy as np import scipy.signal as sg
M, N, P = 4, 10, 20 A = np.random.randn(M, N) B = np.random.randn(M, P)
C = sg.convolve(A, B, 'full')[(2*M-1)/2] ```
Is there a fast way?
- [Repost Q] Need help understanding this code on power consumption prediction using LSTM
Not OP. This question is being reposted to preserve technical content removed from elsewhere. Feel free to add your own answers/discussion.
Original question: Hello everyone!! I am a final year undergrad(electrical engineering) student who just dabbled in machine learning. During our 1 month training period, I chose to do a course in ML/AI and am now seeking to build a project, mainly on electrical energy consumption prediction. I came across this cool code/project(in the link), but cannot understand a word of it ;( Please if anyone of you could spare me some time and explain this code to me..I'll be grateful to you.
What project do you consider good for a beginner, that I can easily explain to others too?? Do you have any ideas?
- [Resource] What's the kernel trick? (explanation)eranraviv.com What is the Kernel Trick?
Every so often I read about the kernel trick. Each time I read about it I need to relearn what it is. Now I am thinking "Eran, don't you have this fancy bl
A nice visualization/example of the kernel trick. A more mathematical explanation can be found here.