Evidence that LLMs are reaching a point of diminishing returns - and what that might mean
Evidence that LLMs are reaching a point of diminishing returns - and what that might mean
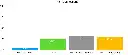
Evidence that LLMs are reaching a point of diminishing returns - and what that might mean

Recent claims that large language model (LLM) capabilities are doubling every 5-14 months appear unfounded based on an analysis of benchmark performance data. While there were huge leaps from GPT-2 to GPT-3 and GPT-3 to GPT-4, the progress from GPT-4 to more recent models like GPT-4 Turbo has been much more modest, suggesting diminishing returns. Plots of accuracy on tasks like MMLU and The New York Times' Connections game show this flattening trend. Qualitatively, core issues like hallucinations and errors persist in the latest models. With multiple models now clustered around GPT-4 level performance but none decisively better, a sense is emerging that the rapid progress of recent years may be stalling out as LLMs approach inherent limitations, potentially leading to disillusionment and a market correction after last year's AI hype.
Summarized by Claude 3 Sonnet